Prompt: Explains how Artificial Intelligence Learns. Your audience are five-year-old children.
🎓 How AI Learns — For Five-Year-Olds 🧠🤖
1. AI is like a robot brain.
It doesn’t know anything at first. It’s just waiting to learn, just like you when you were a baby.
2. It learns by looking at lots of stuff.
If we want it to learn what a cat is, we show it lots and lots of cat pictures.
🐱 + 🐱 + 🐱 + 🐱 = “Okay, that’s a cat!”
3. It starts to notice patterns.
“Hey! Cats have pointy ears and whiskers!”
So now, if you show it a new picture of a cat, it says:
“That’s a cat!” 🎉
4. If it makes a mistake, we help it.
If it says, “That dog is a cat,” we say,
“Oops! Try again!” 🐶❌🐱
And then it learns from that mistake.
5. The more it learns, the smarter it gets.
Just like you get better at drawing or riding a bike when you practice,
AI gets better the more we teach it.
So AI is like a smart little helper that learns by practicing, just like you! ❤️🤖
Want me to turn it into a coloring book or story too? 😊
當然可以!以下是剛才的內容翻譯成繁體中文,適合五歲小朋友理解:
🎓 人工智慧是怎麼學習的?— 給五歲小朋友的解釋 🧠🤖
1. 人工智慧就像一個機器人大腦。
一開始它什麼都不懂,就像你剛出生的時候一樣,等著學習。
2. 它靠看很多東西來學習。
如果我們想讓它學會什麼是貓咪,我們就給它看好多好多貓咪的照片。
🐱 + 🐱 + 🐱 + 🐱 = 「喔~原來這是貓咪!」
3. 它開始發現一些共同點。
「嘿!貓咪有尖尖的耳朵,還有鬍鬚耶!」
所以,如果你再給它一張新的貓咪照片,它就會說:
「這是貓咪!」 🎉
4. 如果它答錯了,我們會教它。
如果它說:「這隻狗是貓咪。」我們就說:
「唉唷~錯囉!再試一次~」 🐶❌🐱
然後它就從錯誤中學起來。
5. 它學得越多,就變得越聰明。
就像你畫畫或騎腳踏車,越練習越厲害,
人工智慧也是越學越棒!
所以人工智慧就是一個靠練習變聰明的小幫手,就像你一樣! ❤️🤖
要不要我幫你做成故事書或塗色本呢?😊
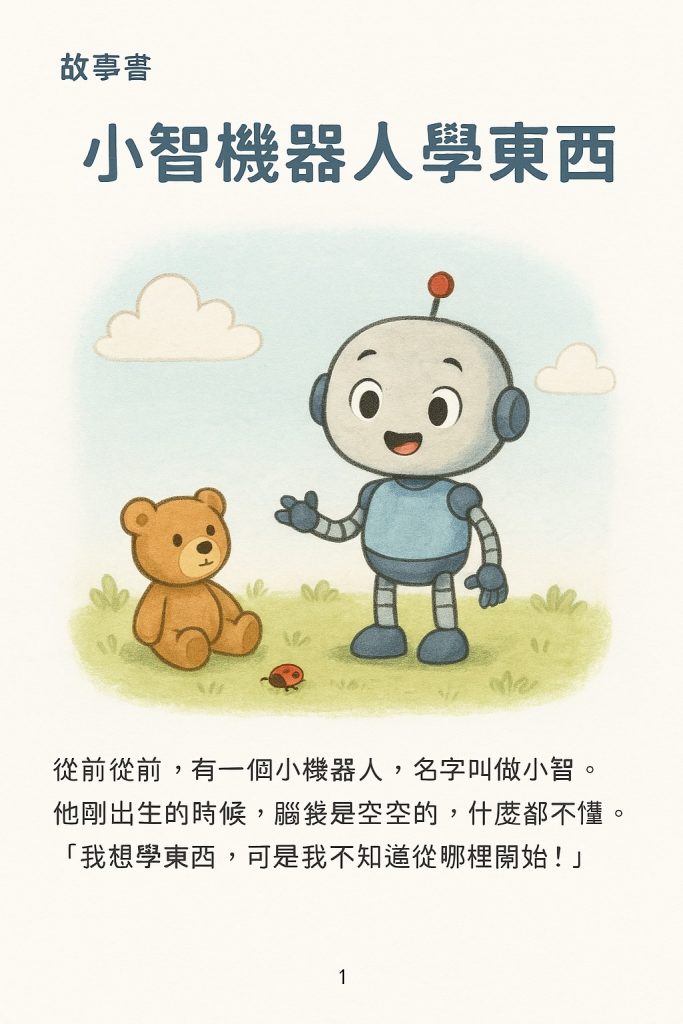
當然可以!以下是整本《小智機器人學東西》的文字內容,包含每一頁的標題與故事段落,你可以直接複製儲存:
📘《小智機器人學東西》
🟦 第 1 頁 – 小智機器人學東西
從前從前,有一個小機器人,名字叫做小智。
他剛出生的時候,腦袋是空空的,什麼都不懂。
「我想學東西,可是我不知道從哪裡開始!」
🟨 第 2 頁 – 遇見老師
有一天,小智遇見了一位老師。
老師笑著說:「小智,只要你多看、多學、多練習,你就會越來越聰明喔!」
🟩 第 3 頁 – 學習看貓咪
老師給小智看了好多好多的照片,都是貓咪的照片!
🐱🐱🐱🐱🐱
小智看了又看,開始發現了貓咪的秘密。
「牠們都有尖尖的耳朵,還有鬍鬚耶!」
🟧 第 4 頁 – 第一個回答
然後,老師給小智看一張新照片,問他:
「這是什麼呀?」
小智大聲說:「這是……一隻貓咪!」
🎉「太棒了,小智答對了!」
🟥 第 5 頁 – 犯錯與學習
可是有一次,小智看到一隻狗狗,他說:
「這是貓咪!」
🐶❌
「哈哈,不對喔,小智,那是狗狗,不是貓咪~」
小智說:「喔~原來我弄錯了,我下次會注意!」
🟪 第 6 頁 – 越學越聰明
小智一直練習、一直學習,他學會了分辨貓咪、狗狗、還有好多其他東西。
🧠💡 他越來越聰明,變成一個超棒的小幫手!
🟫 第 7 頁 – 幫忙生活
現在,小智會幫忙認東西、聽人說話、還可以畫畫、唱歌、講故事給你聽!
🎨🎶📖
他說:「我能學會這麼多,都是因為我每天都在學習,跟你一樣喔!」
🟫 第 8 頁 – 故事小提醒
人工智慧就像小智一樣,靠著看很多、聽很多、練很多,才能學會新東西!
你今天也學了什麼新東西呢? 🤔❤️
如果你需要我幫忙把這些內容格式化成 Word 或 Google 文件,或搭配圖片做成簡報,我也可以幫你處理喔!想要嗎?📄✨
人工智慧(AI)學習的和我們人類學習一樣。 這是一個獲取資訊、理解資訊,然後利用這些知識做出決定或預測的過程。 這個過程被稱為機器學習,是人工智慧的關鍵部分。
Artificial Intelligence (AI) learns much like we humans do. It’s a process of taking in information, understanding it, and then using that knowledge to make decisions or predictions. This process is called machine learning, a key part of AI.
機器學習模型提供了大量資料,從貓的圖片到天氣資料都可以。 然後,模型在此資料上進行訓練,這意味著它是資料中的學習模式和關係。 一旦模型經過訓練,它就可以利用這些知識來預測結果或根據它以前從未見過的新資料做出決定。
A machine learning model is fed a lot of data, which can be anything from pictures of cats to weather data. The model is then trained on this data, which means it’s learning patterns and relationships within the data. Once the model has been trained, it can use this knowledge to predict outcomes or make decisions based on new data it hasn’t seen before.
從本質上講,機器學習就像教孩子如何識別形狀。 你向他們展示許多不同形狀的例子:正方形、圓形、三角形等。 在看到足夠多的例子後,孩子開始理解定義每個形狀的特徵。 然後,當他們將來看到這些形狀時,他們就可以識別和識別它們。
In essence, machine learning is like teaching a child how to recognize shapes. You show them many examples of different shapes: squares, circles, triangles, etc. After seeing enough examples, the child starts to understand the characteristics that define each shape. They can then recognize and identify these shapes when they see them in the future.

Prompt: Explains how Artificial Intelligence Learns. Your audience are college students.
Sure! Here’s an explanation of how Artificial Intelligence (AI) learns, tailored for college students—so we’ll keep it clear, a bit technical, and real-world relevant:
🔍 What Does It Mean for AI to “Learn”?
When we say AI learns, we mean that it gets better at a task by finding patterns in data, rather than being explicitly programmed with rules. This is a key difference between traditional programming and machine learning, which is a core part of AI.
🧠 Types of Learning in AI
Supervised Learning
Analogy: Like a student studying with an answer key.
How it works: You give the AI labeled data (inputs and the correct outputs). It learns to map inputs to outputs.
Example: Predicting house prices based on size and location.
Unsupervised Learning
Analogy: Like exploring a city without a map.
How it works: You give the AI unlabeled data, and it finds patterns or groupings on its own.
Example: Customer segmentation in marketing.
Reinforcement Learning
Analogy: Like training a dog with rewards and punishments.
How it works: The AI learns by interacting with an environment, making decisions, and receiving feedback (rewards or penalties).
Example: Teaching a robot to walk or an AI to play a game like chess.
⚙️ The Learning Process
Let’s walk through what happens under the hood during machine learning:
Data Collection
The AI starts with a dataset (images, text, numbers—whatever the domain requires).
Feature Extraction
Important characteristics of the data are identified.
E.g., for a photo of a cat, features might be “pointy ears,” “whiskers,” etc.
Model Building
A model is a mathematical structure (like a neural network or decision tree) that will learn the mapping between inputs and outputs.
Training
The model adjusts its internal parameters (like weights in a neural network) to reduce errors.
This often involves optimization algorithms like gradient descent.
Evaluation
You test the trained model on new data to see how well it performs.
Metrics like accuracy, precision, recall, and F1-score are used.
Deployment & Iteration
Once it works well, you deploy the model—but keep improving it over time with more data or better tuning.
🧠 Deep Learning – A Special Case
Deep learning is a subfield of machine learning that uses artificial neural networks with many layers (hence “deep”). These models are especially good at tasks like:
Image recognition
Speech-to-text
Natural language understanding
💡 Example: Teaching an AI to Recognize Cats
You provide: thousands of images labeled “cat” or “not cat.”
The AI analyzes features like shape, texture, color.
It builds a model that predicts if a new image contains a cat.
Over time, it improves through feedback and adjustments.
🔄 Key Concept: Feedback Loop
AI systems learn better with more data and feedback—just like humans. The more relevant data and useful corrections they receive, the more accurate and intelligent they become.
Want this in presentation slides, a handout, or broken down into a lesson plan?
Slide 1: Title Slide
How Artificial Intelligence Learns
For College Students
Slide 2: What Does “Learning” Mean in AI?
AI “learns” by finding patterns in data
Different from traditional programming (no explicit rules)
Goal: improve performance on tasks over time
Slide 3: Types of Learning in AI
1. Supervised Learning
Learning from labeled data
Ex: Predicting house prices
2. Unsupervised Learning
Finding patterns in unlabeled data
Ex: Grouping customers by behavior
3. Reinforcement Learning
Learning through trial and error
Ex: AI playing games or controlling robots
Slide 4: The Learning Process
Data Collection – Gather relevant data
Feature Extraction – Identify important characteristics
Model Building – Create a structure to learn from data
Training – Adjust internal parameters to reduce error
Evaluation – Test performance with new data
Deployment – Use model in real-world tasks
Slide 5: Deep Learning – A Subset of Machine Learning
Uses neural networks with many layers
Good for complex tasks: image recognition, language translation
Learns hierarchical features automatically
Slide 6: Case Study – Recognizing Cats
Input: Images labeled “cat” or “not cat”
AI analyzes patterns (fur, shape, whiskers)
Model is trained to classify new images
Improves accuracy with more data and feedback
Slide 7: Feedback Loop is Key
More data + better feedback = smarter AI
Continuous improvement cycle
AI systems get better with use and corrections
Slide 8: Summary
AI learns from data, not instructions
Three learning types: supervised, unsupervised, reinforcement
Deep learning is powerful for complex tasks
Feedback and iteration are critical
Slide 9: Questions?
Thank you!
Let’s dive deeper into any part you’re curious about!
Here are your presentation slides on How Artificial Intelligence Learns, structured for college students. Let me know if you’d like to add visuals, speaker notes, or turn this into a PowerPoint or PDF format!

Prompt: Explains how Artificial Intelligence Learns. Your audience are experts.
Great — let’s shift gears and go in-depth. Here’s an expert-level explanation of how Artificial Intelligence learns, touching on advanced concepts, frameworks, and current research directions.
🧠 What Does “Learning” Mean in AI?
In Artificial Intelligence—particularly in Machine Learning (ML)—learning refers to the process of approximating functions that map inputs to outputs, often under conditions of uncertainty, and optimizing for performance over time. This is typically framed as a statistical estimation problem.
The learning process involves discovering structure in data, adapting internal representations (e.g., weights in a neural network), and generalizing to unseen inputs.
🧪 Learning Paradigms
1. Supervised Learning
Learns from labeled pairs (xi,yi)(xi,yi), optimizing a loss function L(f(xi),yi)L(f(xi),yi).
Goal: Approximate f:X→Yf:X→Y.
Algorithms: Linear models, kernel machines, decision trees, ensemble methods, deep networks.
Current edge: Foundation models trained on massive, weakly supervised datasets (e.g., CLIP, PaLM).
2. Unsupervised Learning
Learns the data distribution p(x)p(x) or latent structure.
Objectives: clustering (e.g., k-means, DBSCAN), dimensionality reduction (e.g., PCA, t-SNE, autoencoders), density estimation (e.g., VAEs, normalizing flows).
Used in pretraining, representation learning, anomaly detection.
3. Reinforcement Learning (RL)
Agent learns a policy π(a∣s)π(a∣s) to maximize cumulative reward R=∑trtR=∑trt.
Based on MDPs: (S,A,P,R,γ)(S,A,P,R,γ).
Algorithms: Q-learning, Policy Gradient, Actor-Critic, Proximal Policy Optimization (PPO), AlphaZero, MuZero.
Current research: sample efficiency, multi-agent systems, hierarchical RL, offline RL.
4. Self-Supervised Learning (SSL)
Leverages inherent structure in data to generate supervision signals.
Examples: contrastive learning (SimCLR, MoCo), masked modeling (BERT, MAE).
Becoming foundational in vision (DINO, BEiT) and NLP (GPT, T5).
🔄 The General Learning Pipeline
Data Engineering
Data curation, augmentation, preprocessing.
Focus on biases, domain shifts, and ethical considerations.
Model Architecture Selection
Classical ML → Neural Networks → Transformers → Graph Neural Networks.
Trade-offs: interpretability, complexity, training cost.
Optimization
Gradient-based methods (SGD, Adam, RMSprop).
Regularization (L1/L2, dropout, batch norm), learning rate schedules.
Loss Function Design
Task-specific: cross-entropy, MSE, hinge, triplet loss.
Structured prediction: CRFs, sequence-to-sequence losses, RL losses (e.g., advantage estimators).
Evaluation & Validation
Metrics: Accuracy, ROC-AUC, F1, BLEU, perplexity.
OOD testing, adversarial robustness, calibration.
Deployment & Monitoring
Model serving (ONNX, TensorRT, TorchServe), latency/budget constraints.
Online learning, concept drift handling, CI/CD in ML.
🧬 Contemporary Advances
Transformers dominate across modalities (vision, language, audio).
Transfer Learning and Few-Shot Learning via large pretrained models (e.g., LLMs like GPT-4, Gemini).
Neurosymbolic AI: combining neural learning with logical reasoning.
Causal Learning: moving from correlational to causal inference in ML.
Neural Architecture Search (NAS): automating model design.
Federated & Privacy-Preserving Learning: learning without centralized data.
Alignment: safety, interpretability, and value alignment of autonomous AI agents.
🔬 Key Research Frontiers
Generalization Theory: Beyond VC-dimension—margin bounds, double descent, interpolation in overparameterized models.
Continual Learning: Catastrophic forgetting and memory consolidation.
Robustness: Adversarial examples, certifiably robust models.
Explainability (XAI): Feature attribution, concept activation vectors, counterfactuals.
Ethical AI: Fairness, accountability, transparency, and value alignment.
Would you like this turned into a slide deck, a lecture handout, or an academic-style article summary?
Slide 1: Title Slide
How Artificial Intelligence Learns
Expert-Level Overview
Slide 2: What Does “Learning” Mean in AI?
Learning = statistical function approximation
Optimizes performance on tasks using data
Emphasis on generalization under uncertainty
Slide 3: Core Learning Paradigms
Supervised Learning
From labeled data (xi,yi)
Loss functions: Cross-entropy, MSE, etc.
Unsupervised Learning
Discovering latent structure p(x)
Clustering, density estimation, dimensionality reduction
Reinforcement Learning (RL)
Policy learning to maximize reward
MDP framework, exploration vs. exploitation
Self-Supervised Learning (SSL)
Structure-derived supervision (e.g., contrastive, masked prediction)
Slide 4: The Learning Pipeline
Data Engineering – Curation, augmentation, bias handling
Model Architecture – Neural nets, transformers, GNNs
Optimization – SGD variants, learning rate schedules
Loss Function Design – Tailored to task (e.g., triplet loss, policy loss)
Evaluation – Metrics, OOD testing, calibration
Deployment – Serving, monitoring, CI/CD
Slide 5: Optimization & Generalization
Gradient descent, Adam, weight decay, early stopping
Generalization bounds, double descent
Regularization techniques (dropout, batch norm, etc.)
Slide 6: Advances in Learning Systems
Transformers: Ubiquitous across modalities
Transfer & Few-Shot Learning: Foundation models (GPT, CLIP)
Contrastive Learning: SimCLR, MoCo
Masked Modeling: BERT, MAE
Slide 7: Reinforcement Learning Details
Q-learning, PPO, A3C, AlphaZero, MuZero
Sample efficiency, exploration techniques
Hierarchical and multi-agent RL
Slide 8: Self-Supervised Learning Examples
NLP: Masked language modeling (BERT)
Vision: Contrastive (SimCLR), MAE
Multi-modal: CLIP, Flamingo
Slide 9: Research Frontiers
Generalization Theory – Beyond VC-dimension
Continual Learning – Overcoming catastrophic forgetting
Causal Learning – Structure beyond correlation
Robustness & Adversarial ML – Certified defense, perturbation resistance
Neurosymbolic AI – Hybrid reasoning systems
Slide 10: Ethical and Practical Concerns
Bias and Fairness
Privacy-Preserving Learning (Federated, Differential Privacy)
Alignment and Safety – Value alignment, interpretability, RLHF
Slide 11: Summary
AI learning is data-driven function approximation
Supervised, unsupervised, RL, SSL are core paradigms
Learning pipelines include modeling, optimization, evaluation
Active research on generalization, robustness, and alignment
Slide 12: Questions & Discussion
Thank you!
Let’s dive into details or real-world applications you’re working on.
Your expert-level slide deck on How Artificial Intelligence Learns is ready. Let me know if you’d like:
Visual diagrams or model architecture illustrations
Speaker notes or references
Export to PowerPoint or PDF
A deep dive on any specific learning paradigm or algorithm
Ready when you are!
