Prompt: "為什麼人工智慧有時候會表現出胡說八道的現象?舉出幾個有趣的實例。"
to #ChatGPT
人工智慧(AI)有時候會表現出「胡說八道」的現象,這通常是由以下幾個因素造成的:
1.機器學習的統計性質
AI 並不是在「理解」內容,而是基於大量數據學習語言模式。當它遇到未見過或模糊的問題時,可能會根據統計關聯性生成看似合理但實際上錯誤的回答。
2.訓練數據的限制
AI 的知識來自訓練數據,如果數據本身有錯誤、偏見或缺乏特定領域的信息,AI 可能會產生錯誤或不準確的回應。
3.缺乏真正的理解與推理能力
AI 沒有真正的「理解」,它只是根據詞語的關聯性生成回應,而不是像人類一樣有邏輯推理或常識判斷能力。因此,在某些情境下,它可能會組合出毫無根據的內容。
4.過度一般化或錯誤推斷
AI 可能會基於有限的數據推斷出錯誤的規律,導致回答出現不合理的推論。例如,它可能會把兩個相似但無關的概念混為一談。
5.隨機性(溫度參數)
AI 的回答通常帶有一定程度的隨機性,特別是在溫度(temperature)參數較高時,會更傾向於產生創意性或隨機的回應,但這也可能導致「胡說八道」的現象。
6.缺乏即時更新能力
如果 AI 沒有即時連接到最新資訊,它的回答可能會基於過時的知識,從而出錯。
這些問題目前仍是 AI 領域正在努力解決的挑戰,因此在使用 AI 回答時,仍需要人類的判斷與驗證。
當 AI 胡說八道時,可能會產生一些有趣甚至荒謬的錯誤,這類現象在 AI 研究領域被稱為 「幻覺」(hallucination)。以下是幾個經典的實例:
1. 偽造的學術論文與文獻
AI 生成模型有時會在回答學術問題時捏造不存在的論文。例如,當被要求提供某個主題的參考文獻時,它可能會編造出一篇看似合理的論文,甚至包含假作者、假標題和假發表期刊。這些內容看似真實,但實際上根本不存在!
2. 鬼才數學答案
有些 AI 會給出數學問題的荒謬解法,例如:
•問:「如果我有兩個蘋果,拿走一個,還剩幾個?」
•AI:「你現在有 1.5 個蘋果,因為量子力學告訴我們物體可以處於疊加狀態。」
這種回答雖然聽起來有創意,但完全偏離了正確答案。
3. 胡亂解釋歷史事件
AI 可能會錯誤地組合歷史資訊,例如:
•AI 曾回答:「拿破崙和亞伯拉罕·林肯在二戰期間合作制定了《凡爾賽條約》。」
這完全是胡扯,因為林肯和拿破崙分屬不同時代,而凡爾賽條約則屬於一戰後的產物。
4. 錯誤的生物學知識
AI 在生物學領域也可能產生幻覺,例如:
•問:「鴕鳥能飛嗎?」
•AI:「當然!鴕鳥的翅膀特化為超音速飛行,因此能夠在短時間內突破音障。」
這顯然是錯的,鴕鳥是不能飛的,但 AI 可能會根據某些動物的特徵錯誤推斷。
5. 胡亂翻譯與詞義錯誤
在翻譯時,AI 可能會產生讓人哭笑不得的錯誤。例如,某些早期翻譯 AI 曾將「Out of sight, out of mind」(眼不見,心不煩) 翻譯成 「盲人的腦袋壞掉了」,完全曲解了原意。
6. 瞎編名人語錄
如果你問 AI:「愛因斯坦說過什麼鼓舞人心的話?」它可能會生成:「愛因斯坦曾說:『人生就像騎自行車,要保持平衡就得繼續前進。』」這句話聽起來很合理,但實際上沒有證據顯示愛因斯坦真的說過這句話。
這些例子展示了 AI 生成內容的局限性,也提醒我們在使用 AI 時,應該保持批判性思考,避免盲目信任其回答。
Why Does AI Sometimes Produce Nonsensical Responses?
Artificial intelligence (AI) sometimes produces nonsensical responses due to several key reasons:
1.The Statistical Nature of Machine Learning
AI does not “understand” content in the way humans do; it learns language patterns based on vast amounts of data. When faced with unfamiliar or ambiguous questions, it may generate responses that seem reasonable but are actually incorrect.
2.Limitations of Training Data
AI’s knowledge is based on its training data. If the data contains errors, biases, or lacks information in a specific domain, the AI may produce inaccurate or misleading answers.
3.Lack of True Understanding and Reasoning
AI lacks genuine comprehension and logical reasoning. Instead of thinking like humans, it generates responses based on word associations, which can sometimes lead to baseless or nonsensical content.
4.Overgeneralization or Incorrect Inferences
AI may mistakenly infer patterns from limited data, leading to incorrect conclusions. For example, it might mix up two similar but unrelated concepts.
5.Randomness (Temperature Parameter)
AI responses often include some level of randomness, especially when the temperature setting is high. This randomness can encourage creativity but may also cause the AI to produce incoherent or incorrect statements.
6.Lack of Real-Time Updates
If an AI system does not have access to real-time information, its responses may be outdated or incorrect based on old knowledge.
These challenges remain ongoing issues in AI research, highlighting the importance of human oversight and verification when using AI-generated responses.
Interesting Examples of AI Hallucinations
When AI produces nonsensical answers, it can sometimes create amusing or absurd errors. These cases are known as “hallucinations” in AI research. Here are some classic examples:
1. Fabricated Academic Papers and References
AI models sometimes generate entirely fake academic papers. When asked for references on a topic, an AI might produce a scholarly-looking citation, including a title, author, and journal name—all of which do not exist. The citation appears legitimate but is entirely fabricated!
2. Bizarre Math Answers
Some AI models give hilariously incorrect math explanations. For example:
•Question: “If I have two apples and take one away, how many do I have left?”
•AI: “You now have 1.5 apples because quantum mechanics tells us that objects can exist in a superposition state.”
While creative, this answer is completely incorrect.
3. Incorrect Explanations of Historical Events
AI can sometimes mix up historical facts. For example:
•AI once claimed: “Napoleon and Abraham Lincoln collaborated during World War II to draft the Treaty of Versailles.”
This is entirely false—Napoleon and Lincoln lived in different eras, and the Treaty of Versailles was a post-World War I agreement.
4. False Biological Facts
AI sometimes makes bizarre claims about biology. For instance:
•Question: “Can ostriches fly?”
•AI: “Of course! Ostriches’ wings are adapted for supersonic flight, allowing them to break the sound barrier within seconds.”
Ostriches are flightless birds, but the AI incorrectly extrapolated information based on certain animal traits.
5. Absurd Translation Errors
Early AI translation systems made some laughable mistakes. One classic example is translating “Out of sight, out of mind” (meaning “if you don’t see something, you won’t think about it”) into “Blind people have broken brains.” This is a complete misunderstanding of the phrase.
6. Fake Quotes from Famous Figures
If you ask AI, “What are some inspiring quotes from Albert Einstein?” it might respond:
•“Einstein once said, ‘Life is like riding a bicycle. To keep your balance, you must keep moving.’”
This quote is commonly attributed to Einstein, but there is no solid evidence he ever said it. AI sometimes fabricates or misattributes quotes to well-known figures.
These examples illustrate AI’s limitations and serve as a reminder that we should always fact-check its responses rather than blindly trusting them.
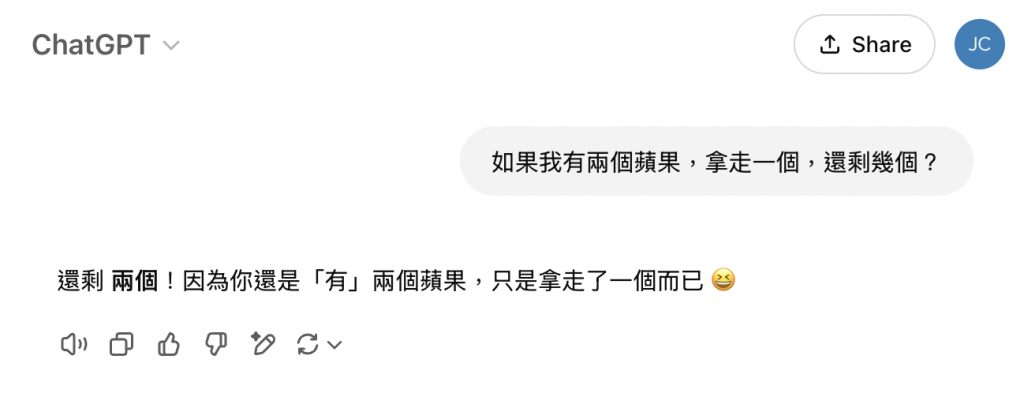
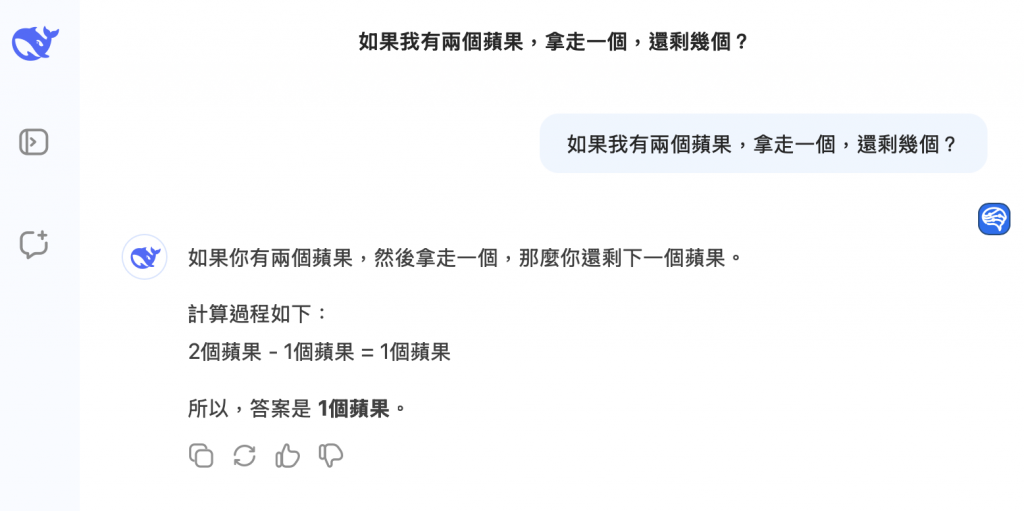

Prompt: How can we improve the prompt statement to reduce hallucinations in AI?
以下是減少AI生成內容幻覺(Hallucination)的提示語優化策略,翻譯為繁體中文:
減少AI幻覺的提問優化策略(繁體中文版)
提高具體性
範例:將「解釋氣候變遷」改為「列出過去五年中三項關於人類對氣候變遷影響的同行評審研究」。
原因:縮小範圍可減少虛構可能性。
要求證據導向
用語:加入「引用可信來源」或「使用[特定資料庫/日期]的數據」。
原因:將回答錨定於事實依據。
鼓勵坦承不確定性
用語:明確要求「若不確定,請回答『我不知道』,而非猜測」。
原因:避免資訊不足時的臆測。
分步驟推理
範例:指示「先概述X的成因,再根據[來源A]和[來源B]提供證據」。
原因:分解複雜問題,降低錯誤率。
提供示例(少量樣本學習)
範例:先給出正確回答範本供模型模仿。
原因:引導結構與語氣,減少偏差。
設定明確限制
用語:如「限100字內回答」或「避免主觀意見,聚焦事實」。
原因:抑制無關或過度「創作」內容。
引用用戶提供的資料
範例:明確寫「根據以下文本[貼上文本],總結⋯⋯」。
原因:直接將回答綁定於指定資訊。
迭代式澄清
做法:追問如「請根據[來源]驗證此說法」。
原因:修正初始回答的潛在錯誤。
避免開放式問題
改寫範例:將「討論AI倫理」改為「根據2023年IEEE指南,AI領域三大倫理顧慮為何?」
原因:具體問題限制無關內容。
要求標註知識缺口
用語:加入「若資料不足,請說明缺失部分」。
原因:促使模型坦承限制,而非編造。
注意事項
平衡取捨:過度限制可能降低實用性,需測試不同提示語以最佳化效果。
本質限制:即使優化提示語,AI幻覺無法完全消除,關鍵內容仍需人工驗證。
透過上述結構化調整,可顯著提升AI回答的可信度與精準度。
To reduce hallucinations in AI responses, refine your prompts using the following structured strategies:
Enhance Specificity:
Example: Instead of “Explain climate change,” use “List three peer-reviewed studies from the last five years on human impact on climate change.”
Why: Narrow focus reduces room for invention.
Demand Evidence-Based Responses:
Phrase: “Cite verified sources” or “Use data from [specific repository/date].”
Why: Anchors the response in factual content.
Encourage Uncertainty Disclosure:
Phrase: “If unsure, state ‘I don’t know’ instead of guessing.”
Why: Prevents speculative answers when information is lacking.
Step-by-Step Reasoning:
Example: “First, outline the causes of X. Next, provide evidence from [Source A] and [Source B].”
Why: Breaks down complexity, reducing errors.
Leverage Examples (Few-Shot Learning):
Example: Provide a correct response template for the model to mimic.
Why: Guides structure and tone, minimizing deviations.
Set Explicit Constraints:
Phrase: “Respond in 100 words or less” or “Avoid opinions; focus on facts.”
Why: Limits tangential or creative outputs.
Reference User-Provided Data:
Phrase: “Based on the text provided [paste text], summarize…”
Why: Ties answers directly to supplied information.
Iterative Clarification:
Approach: Ask follow-ups like, “Verify this against [Source].”
Why: Allows correction of initial inaccuracies.
Avoid Open-Ended Queries:
Instead of: “Discuss AI ethics,” ask “What are three key ethical concerns in AI per the 2023 IEEE guidelines?”
Why: Specificity curtails irrelevant content.
Acknowledge Model Limits:
Phrase: “If data is insufficient, clarify gaps.”
Why: Prompts transparency over fabrication.
Balancing Act: Over-constraining may stifle usefulness. Test variations to optimize clarity and relevance. While these methods reduce hallucinations, they may not eliminate them entirely—critical evaluation of outputs remains essential.
